Washington, DC—A team of scientists led by Carnegie’s Robert Hazen and visiting scholar Jim Cleaves of the Tokyo Institute of Technology and the Blue Marble Space Institute for Science have discovered what they’re calling the “holy grail of astrobiology,” a simple and reliable test for signs of past or present life on other planets. Their findings are published in Proceedings of the National Academy of Sciences.
The research team—which included Carnegie’s Anirudh Prabhu, Michael Wong, and George Cody, as well as Grethe Hystad of Purdue University and Sophia Economon of Johns Hopkins University—report that, with 90 percent accuracy, their artificial intelligence-based method distinguished modern and ancient biological samples from those of abiotic origin.
Simply put, the new test reliably determines whether the history of a sample under examination included something that was once alive.
“This routine analytical method has the potential to revolutionize the search for extraterrestrial life and deepen our understanding of both the origin and chemistry of the earliest life on Earth,” Hazen said. “It opens the way to using smart sensors on robotic spacecraft, landers and rovers to search for signs of life before the samples return to Earth.”
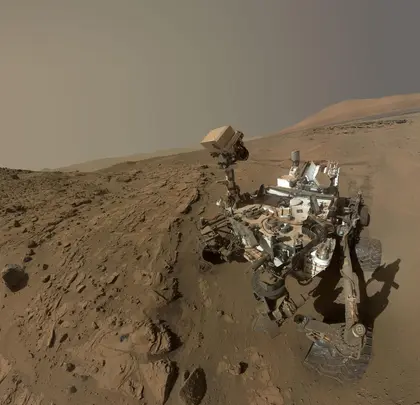
Most immediately, the new test could reveal the history of mysterious, ancient rocks on Earth, and possibly that of samples already collected by the Mars Curiosity rover’s Sample Analysis at Mars (SAM) instrument.
“The search for extraterrestrial life remains one of the most tantalizing endeavors in modern science,” says lead author Cleaves. “The implications of this new research are many, but there are three big takeaways: First, at some deep level, biochemistry differs from abiotic organic chemistry; second, we can look at Mars and ancient Earth samples to tell if they were once alive; and third, it is likely this new method could distinguish alternative biospheres from those of Earth, with significant implications for future astrobiology missions.”
The innovative analytical method does not rely simply on identifying a specific molecule or group of compounds in a sample. Instead, the researchers demonstrated that AI can differentiate biotic from abiotic samples by detecting subtle differences within a sample’s molecular patterns as revealed by pyrolysis gas chromatography analysis—which separates and identifies a sample’s component parts—followed by mass spectrometry—which determines the molecular weights of those components.
Vast multidimensional data from the molecular analyses of 134 known abiotic or biotic carbon-rich samples were used to train AI to predict a new sample’s origin. With approximately 90 percent accuracy, AI successfully identified samples that had originated from:
- Living things, such as modern shells, teeth, bones, insects, leaves, rice, human hair, and
cells preserved in fine-grained rock;
- Remnants of ancient life altered by geological processing (e.g. coal, oil, amber, and
carbon-rich fossils); or
- Samples with abiotic origins, such as pure laboratory chemicals (e.g., amino acids) and
carbon-rich meteorites.

The authors add that until now the origins of many ancient carbon-bearing samples have been difficult to determine because collections of organic molecules, whether biotic or abiotic, tend to degrade over time. Surprisingly, in spite of significant decay and alteration, the new analytical method detected signs of biology preserved in some instances over hundreds of millions of years.
“We began with the idea that the chemistry of life differs fundamentally from that of the inanimate world; that there are ‘chemical rules of life’ that influence the diversity and distribution of biomolecules,” Hazen said. “If we could deduce those rules, we can use them to guide our efforts to model life’s origins or to detect subtle signs of life on other worlds.”
Prabhu explained the role of A.I. by talking about the idea of separating coins using different attributes—monetary value, metal type, year of minting, weight, or radius, for example—then going further to find combinations of attributes that create more nuanced separations and groupings.
“And when hundreds of such attributes are involved, A.I. algorithms are invaluable to collate the information and create highly nuanced insights,” he said.
The technique may soon resolve a number of scientific mysteries on Earth, including the origin of 3.5-billion-year-old black sediments from Western Australia—hotly debated rocks that some researchers contend hold Earth’s oldest fossil microbes, while others claim are devoid of life signs.
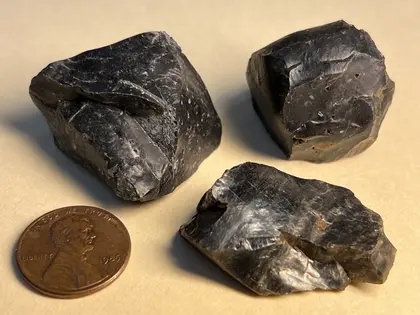
Other samples from ancient rocks in Northern Canada, South Africa, and China evoke similar debates.
“We’re applying our methods right now to address these long-standing questions about the biogenicity of the organic material in these rocks,” Hazen said.
And new ideas have poured forth about the potential contributions of this new approach in other fields such as biology, paleontology, and archaeology.
“If A.I. can easily distinguish biotic from abiotic, as well as modern from ancient life, then what other insights might we gain? For example, could we tease out whether an ancient fossil cell had a nucleus, or was photosynthetic?” Hazen concludes. “Could it analyze charred remains and discriminate different kinds of wood from an archeological site? It’s as if we are just dipping our toes in the water of a vast ocean of possibilities.”
Acknowledgments
This work was funded by the John Templeton Foundation.